How the composition of Tesla’s autopilot software gives clues to how we should invest, recognizing there are no perfect algorithms for driving or investing.
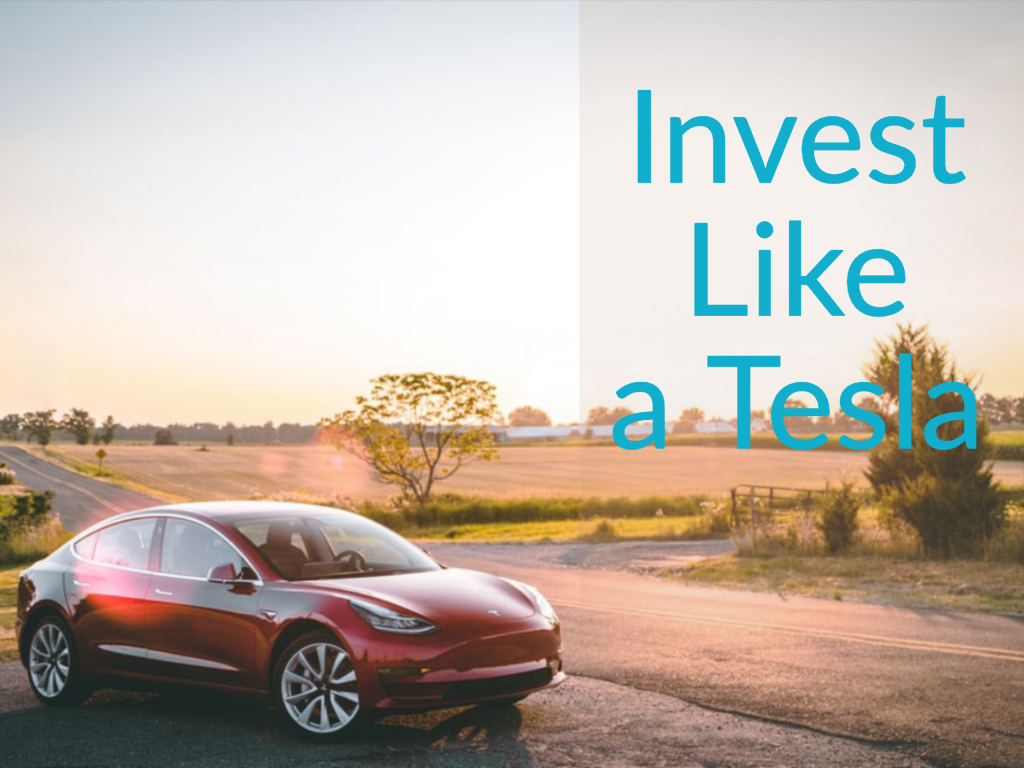
In this episode you’ll learn:
- Why Americans are afraid of self-driving cars.
- How autonomous automobile software works.
- Why people reject even the best possible algorithms.
- What are examples of safety features and rules of thumb we should build into our investing process.
- Why does everyone think a recession is coming soon even though there is little evidence currently.
Show Notes
Brookings survey finds only 21 percent willing to ride in a self-driving car by Darrell M. West
Automated Vehicles for Safety—National Highway Traffic Safety Administration
How do driverless cars work? by James Armstrong—The TelegraphPeople Reject Even the Best Possible Algorithm in Uncertain Decision Domains by Berkeley Dietvorst and Soaham Bharti
Want Less-Biased Decisions? Use Algorithms by Alex P. Miller—Harvard Business Review
Markets are braced for a global downturn—The Economist
Tweet by Ben Carlson posted on Aug 16, 2019
Episode Sponsors
Episode Summary
Should you trust algorithmic decision making when you invest? Many people don’t trust artificial intelligence to make decisions for them, including trusting a self-driving vehicle to safely navigate traffic for them. In this episode of Money For the Rest of Us, David compares trusting algorithms in investing to driving a Tesla; letting go of control could be the safest and most accurate answer.
Fear of algorithms and the search for the perfect answers
According to the Brookings Institute, only 21% of adult internet users said that they would be inclined to trust a self-driving car. Why such a low percentage? 94% of accidents occur due to human error. Automated vehicles are simply the next logical step towards a safer transportation system, yet the majority of drivers say they would never try one. David explains that the fear of algorithms—of letting AI take control—is rooted in humans’ desire for the perfect answer. We somehow believe that we will have greater adaptability and skills to meet trouble or a problem than algorithms will. The statistics, however, demonstrate a different story. Many algorithms are more accurate than humans—including the algorithms used in automated vehicles such as Tesla. The reality is, we will never find the perfect answer or a perfect solution. Even so, people are scared of letting the best possible answer be the one they trust—algorithmic decision making.
Algorithmic decision making is more trustworthy than human decision making
In circumstances of irreducible uncertainty, we are more likely to choose the method that we believe has the greater capacity to be 100% correct vs. the method that is proven to be correct most of the time. We can’t hold algorithms to the standard of perfection. But we can’t hold ourselves to that standard either. We have to be willing to trust the solution that offers the best answer, the best guardrails, and the best safety mechanisms. Instead of asking whether or not algorithms are perfect, we should be asking if they are better than the status quo—human adaptability and decision making.
Testing has proven that algorithms are consistently more accurate and less biased than humans. They are being used heavily in the medical field, as well as in judiciary systems, recruiting, and mortgage applications. Where humans are often biased, the algorithms used are consistently less so. As long as algorithms provide us with the guardrails we need in driving and investing, they shouldn’t be something to fear or avoid. They should be utilized for the user’s best advantage.
Anxiety is the permeating characteristic of today’s market
Many fear that a recession is coming, but there is little evidence to suggest its looming arrival. David encourages listeners to consider the ways they can build guardrails for themselves to create strong portfolios that can stand the test of a possible recession. While algorithms can certainly help, even a Tesla isn’t completely driven by its own system. The driver has control over how much control the vehicle has. The same concept applies to investing.
Recently, the yield curve in the U.S. went negative. Many were frightened by the event, saying a recession is on its way. While storm clouds may be brewing, it doesn’t mean that a recession is inevitable. In fact, there is little to suggest it will materialize. There are still plenty of job opportunities, credit is easy, and oil remains cheap. We don’t need to be all-reliant upon human predictions. Yes, we need guardrails in our investing strategies, but humans cannot predict the future, and we shouldn’t place our trust in what we cannot accomplish.
Invest within the guardrails and diversify your return-drivers
While there is no perfect answer, there are safety features that we can take advantage of in our investing. Just as Tesla isn’t foolproof against disaster, no investment algorithm will be either. But both have safety mechanisms built in that allow for more accurate actions than the human equivalent.
What can you do as you continue to invest? David suggests understanding return drivers—what an investment’s cash flow is, how is the cash flow expected to grow over time, and what investors are paying for the cashflow. Another guardrail against anxious investing is to diversify your portfolio and cash flow streams. Some of your return drivers will disappoint, but others will do very well—allowing you peace of mind in a balanced portfolio. David explains that having multiple streams of income is similar to planting a garden. No one wants to plant an entire garden with one type of flower. Instead, a variety of flowers are usually planted, allowing for a greater opportunity of success. Be sure to listen to the entire episode for more insight into investing like a Tesla and letting go of anxiety in your investing.
Episode Chronology
- [0:17] The pervading fear of self-driving cars, despite their safety features.
- [3:33] Why do people fear algorithms and prefer human decision-making?
- [7:45] Algorithmic decision-making has proven to be most accurate.
- [10:10] Automating your investing is like choosing an automated vehicle.
- [12:06] Keeping within the guardrails of investing strategy.
- [15:44] How to diversify your portfolio as an additional guardrail.
- [17:31] Is a recession really looming on the horizon?
- [20:16] Don’t maximize for perfect answers.
Related Episodes
314: How to Not Have a Lost Decade
Transcript
Welcome to Money For The Rest of Us. This is a personal finance show on money, how it works, how to invest it, and how to live without worrying about it. I’m your host David Stein. Today is episode 265. It’s titled “Invest Like A Tesla.” A few weeks ago I test drove a Tesla Model 3. I was in San Diego. We went out on the highway and we turned on autopilot.
I was a little freaked out. I’ve had a car that has had adaptive cruise control. I was comfortable with that, but the idea of the car changing lanes, letting go of the steering wheel to allow it to change lanes. I think you let go of the steering wheel, at least yet you held onto it and it drove for you. But it didn’t completely drive for you and so it was sort of how much control should I give this car?
The Brookings Institute did a survey last year and found that only 21% of adult internet users said they are inclined to use a self-driving car. 61% said they would never do it. The American Automobile Association survey, this is from this past March, said 71% of those that responded feared autonomous vehicles. Greg Brandon, who’s the AAA director of automotive engineering said, “It’s possible that the sustained level of fear is rooted in a heightened focus, whether good or bad on incidents involving these types of vehicles.” Self-driving cars. And when we’ve been down in Phoenix, you see them, particularly in the Chandler area, Waymo, there’s self-driving cars being tested.
Car safety
The National Highway Transportation Safety Association says 94% of accidents are from human error. Now that seems low to me because there aren’t that many self-driving cars on the road. Cars typically don’t just take off in terms of their acceleration. It’s human error, it’s distractions, it’s texting while driving. It’s fatigue, it’s falling asleep, it’s being overly aggressive when in a hurry, or it’s just making a mistake in not seeing a car and you pull out in front of it.
Autonomous vehicles are essentially an extension of safety features. More and more safety features are being added to cars. This Prius we just got has sensors all over the place. It will bump you back into your lane if you cross over the line. Essentially a self-driving car is just a bundle of safety features. James Armstrong wrote an article in the Telegraph about what is a self-driving car, and he points out “radar sensors around the car monitor the position of vehicles nearby. Video cameras detect traffic lights, read road signs, and keep track of other vehicles, while also looking out for pedestrians and other obstacles. Lidar sensors help to detect the edges of the road and identify lane markings by bouncing pulses of light off the cars surrounding. Ultrasonic centers in the wheels can detect the position of the curb and other vehicles when parking.” And then finally he writes, “A central computer analyzes all of the data from the various centers to manipulate the steering, acceleration, and braking.”That’s what a self-driving car is and we’re terrified of the thought. I was terrified to let these sensors do their job.
The fear of algorithms
There’s a fascinating paper I found this week that explains why we’re afraid to let algorithms work for us, even though the algorithms can do a better job. Berkeley Dietvorst and Soaham Bharti of the University of Chicago, their paper is titled “People Reject Even the Best Possible Algorithm in Uncertain Decision Domains.”
One of the phrases that just stood out to me in reading the paper is this, “People want to maximize the likelihood of perfect answers.” We want algorithms to be perfect. When we think about Tesla, we focus on those few times that the Tesla autopilot and other self-driving cars resulted in a driver or a pedestrian death, but we ignore that the average number of errors for a self-driving car is significantly less on a per car basis than it is for a human driving a car.
In their paper, the authors write, “We propose that decision-makers often have the goal of maximizing their probability of providing a perfect answer. In other words, avoiding any error instead of, for example, minimizing their average level of error. As a result, when a decision-maker is choosing between two decision-making methods, they select the method that they believe to have the higher probability of providing a perfect answer instead of the method that performs better than average.”
Why do we do that? Well, we do it in the face of irreducible uncertainty. In other words, where we don’t really know what’s going to happen and we don’t know what’s going to happen until after it does. Investing is like that, completely uncertain. We’ve talked about it being a complex adaptive system where all the players are adapting and learning over time. In that scenario, a human decision-maker is not going to be right all the time, nor is an algorithm.
Now, if you contrast that with deterministic domains where there is no irreducible uncertainty, solving a math problem, you know that’s going to be solved. And so we trust a calculator because there’s just one answer and the calculator can do it faster than us and it’ll give a perfect answer. But in uncertain domains where there’s a huge amount of uncertainty, we don’t trust algorithms because, and this is their theory because we want the algorithm to be perfect. We hold the algorithm to a higher standard than we hold ourselves.
And in this quest for perfection, they suggest that we actually prefer humans even though they’re wrong more often. In other words, greater variability in their responses. Sometimes they’re correct. Often they’re wrong. We tend to overemphasize the times that they’re correct and de-emphasize when they’re wrong, but when looking out into the future and we don’t know what the answer is, we trust humans more because we think they’re more flexible. That algorithm’s constrained and is less likely to get a perfect answer.
It’s an interesting theory. They write, “A chooser who only considers each method’s likelihood of being perfect will value the upside of higher variance, increased probability of perfect answers, and underweight the downside, i.e. increased probability of terrible answers. Thus such a decision-maker may see high variance a positive attribute.” They say, “The flexibility around the human’s future strategy will maintain the possibility that they could be more likely to provide perfect forecast in the future.” We think humans are adaptable, they are adaptable, and we trust them more because we believe that maybe this time they’ll get it right.
As a Money For the Rest of Us Plus member, you are able to listen to the podcast in an ad-free format and have access to the written transcript for each week’s episode. For listeners with hearing or other impairments that would like access to transcripts please send an email to jd@moneyfortherestofus.com Learn More About Plus Membership »